Cloud and edge computing
02
Edge computing: processing at the source
Edge computing is revolutionizing the way data is processed by bringing capacity and performance closer to where it’s needed. It significantly reduces latency, enhances performance, and enables real-time applications that were previously impossible. Driven by AI, 5G connectivity, and decentralized cloud systems, edge computing is unlocking the potential of technologies ranging from autonomous vehicles to precision healthcare and smart cities.
And yet challenges remain: Security vulnerabilities, managing data across distributed networks, and ensuring seamless integration with centralized cloud systems are critical issues that need to be covered to unlock the full potential.
And yet challenges remain: Security vulnerabilities, managing data across distributed networks, and ensuring seamless integration with centralized cloud systems are critical issues that need to be covered to unlock the full potential.
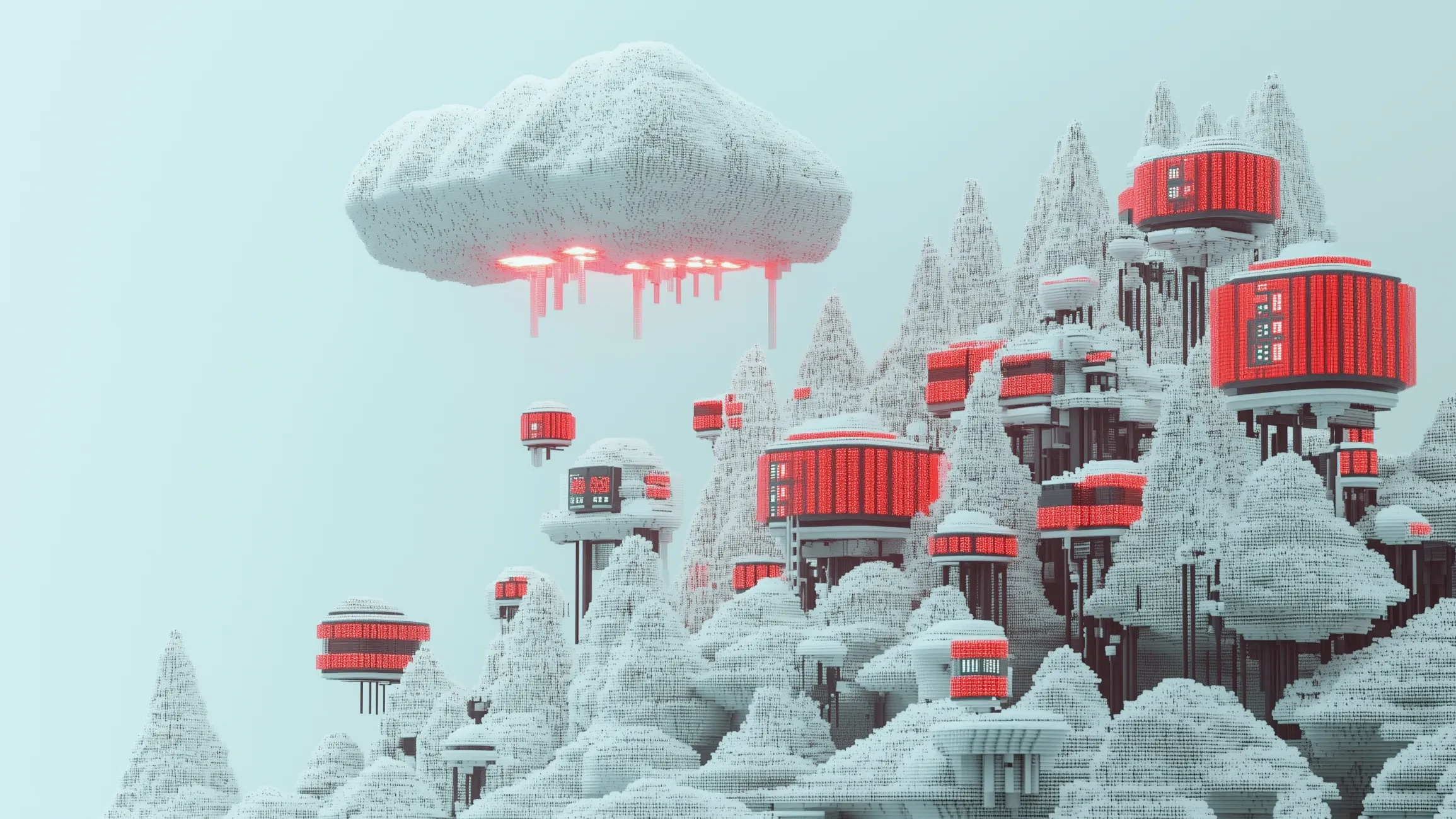
Now: Edge connectivity and efficiency
Edge computing is already delivering on the promise of reducing latency and increasing efficiency. By processing data closer to its source – whether through edge gateways, local processing nodes, or device-embedded intelligence – businesses can minimize the delays associated with transmitting data to distant data centers. These solutions enable real-time response in applications such as industrial automation, gaming, and IoT-driven logistics, where milliseconds matter.
Cloud integration for ISPs: Internet Service Providers (ISPs) are turning to edge computing to provide scalable, integrated solutions that improve the user experience. For instance, content delivery networks (CDNs) use edge nodes to enable faster video streaming and shorter buffering times. However, balancing edge and cloud offerings poses challenges for ISPs, including high costs of upgrading infrastructure, navigating regulatory requirements, and ensuring consistent service quality across regions.
Edge in multi-cloud environments: Edge computing is also driving innovation in multi-cloud solutions. Companies are increasingly integrating services from multiple cloud providers into localized environments controlled by Tier 2 and Tier 3 data centers. This approach improves interconnectivity, ensures data sovereignty, and supports more robust disaster recovery strategies. However, the seamless management of these hybrid environments requires sophisticated orchestration tools to unify different cloud platforms while maintaining operational efficiency.
Cloud integration for ISPs: Internet Service Providers (ISPs) are turning to edge computing to provide scalable, integrated solutions that improve the user experience. For instance, content delivery networks (CDNs) use edge nodes to enable faster video streaming and shorter buffering times. However, balancing edge and cloud offerings poses challenges for ISPs, including high costs of upgrading infrastructure, navigating regulatory requirements, and ensuring consistent service quality across regions.
Edge in multi-cloud environments: Edge computing is also driving innovation in multi-cloud solutions. Companies are increasingly integrating services from multiple cloud providers into localized environments controlled by Tier 2 and Tier 3 data centers. This approach improves interconnectivity, ensures data sovereignty, and supports more robust disaster recovery strategies. However, the seamless management of these hybrid environments requires sophisticated orchestration tools to unify different cloud platforms while maintaining operational efficiency.
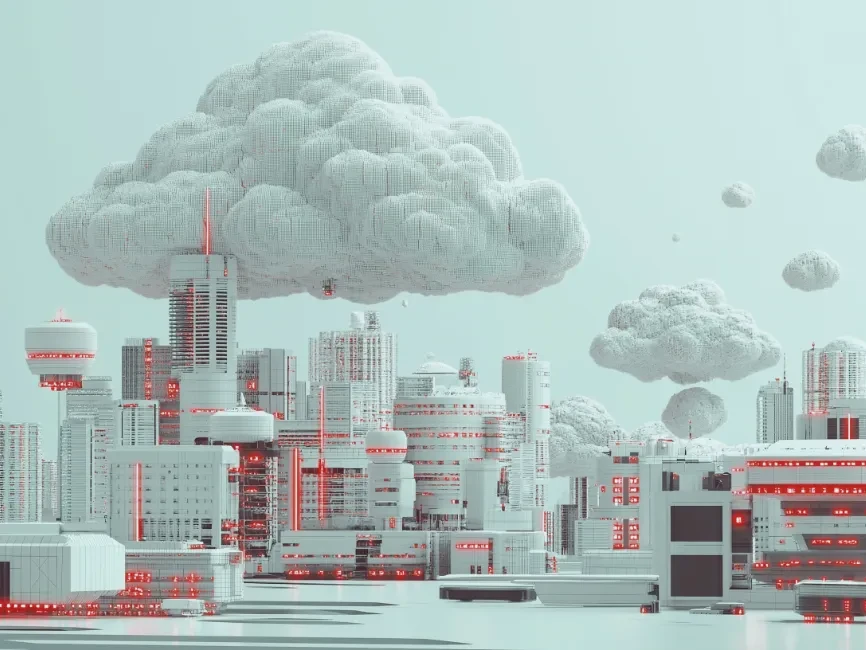
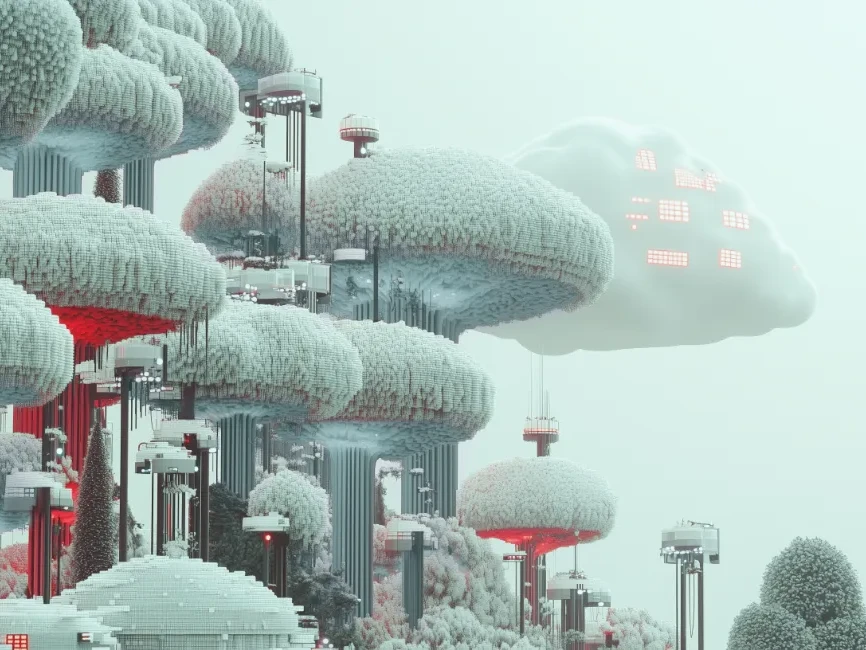
Next: Revolutionizing AI training with decentralized data processing
With the increasing complexity of AI systems, the decentralization of data processing tasks is becoming indispensable. Future AI training will lean heavily on disaggregated, distributed infrastructures that combine cloud and edge computing. This setup will overcome the physical limitations of traditional data centers and allows AI models to train in extensive urban networks. Ultra Ethernet will play a critical role in this evolution, providing the high-speed, low-latency connectivity required to bridge distances between distributed AI infrastraucture. By processing workloads closer to their data sources, edge computing will optimize resource usage, reduce bottlenecks, and enable faster, more efficient AI training. This trend signals a move towards AI ecosystems that are not only scalable, but also more adaptable to localized demands.
Humanoid robots in factories and homes: The next wave of robotics will see humanoid robots deployed in industry and homes, with cloud-edge continuum forming the backbone of their adaptability. These robots will use cloud-based platforms to download and share skills, enabling continuous updates and dynamic learning. Edge computing will enhance their ability to respond to real-time signals by allowing them to process immediate data locally, while seamlessly integrating new knowledge from the cloud. In factories, this will lead to smarter robots capable of handling complex workflows and adjusting to unexpected scenarios without delay. In private households, humanoid robots will provide personalized assistance, responding instantly to individual household needs. These robots will become indispensable companions in both professional and personal spaces, combining responsiveness with continuous learning.
Provocative Consideration: The convergence of these technologies challenges traditional centralized computing models, suggesting a future where processing is ubiquitously distributed. Alongside data centers and clouds of all shapes and sizes and devices with varying potential for edge processing, it will be the networks and interconnection services that bridge the gaps between the edge and larger, centralized processing and storage capabilities. The cloud-edge continuum demands low-latency, stable, and secure connectivity.
Humanoid robots in factories and homes: The next wave of robotics will see humanoid robots deployed in industry and homes, with cloud-edge continuum forming the backbone of their adaptability. These robots will use cloud-based platforms to download and share skills, enabling continuous updates and dynamic learning. Edge computing will enhance their ability to respond to real-time signals by allowing them to process immediate data locally, while seamlessly integrating new knowledge from the cloud. In factories, this will lead to smarter robots capable of handling complex workflows and adjusting to unexpected scenarios without delay. In private households, humanoid robots will provide personalized assistance, responding instantly to individual household needs. These robots will become indispensable companions in both professional and personal spaces, combining responsiveness with continuous learning.
Provocative Consideration: The convergence of these technologies challenges traditional centralized computing models, suggesting a future where processing is ubiquitously distributed. Alongside data centers and clouds of all shapes and sizes and devices with varying potential for edge processing, it will be the networks and interconnection services that bridge the gaps between the edge and larger, centralized processing and storage capabilities. The cloud-edge continuum demands low-latency, stable, and secure connectivity.