Three things to consider as you build your AI data center strategy
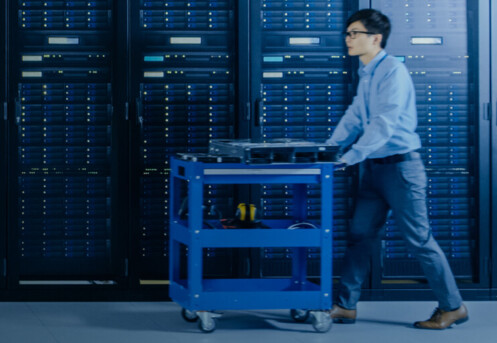
Artificial intelligence (AI) is set to have an immense impact on business productivity. Research from McKinsey & Company estimates generative AI alone could add the equivalent of 4.4 trillion USD in productivity gains to the global economy each and every year. As these technologies become more accessible, it’s no wonder enterprise AI initiatives are shifting from a nice-to-have to a core element of enterprise strategy.
Most of these AI initiatives are taking place in the cloud, where businesses benefit from speed, accessibility, scalability, and security. But just because enterprises are making use of cloud environments, that doesn’t mean they don’t need to pay attention to the connectivity infrastructure powering their AI endeavors. They need to ensure cloud connectivity is robust, resilient, and lightning fast, with short, direct data pathways and quick reaction times. One way to achieve this is to have a solid AI data center strategy in place.
Here are three factors to consider when you’re building an AI-ready enterprise data center strategy.
1. Scalability and processing power
Due to the enormous volumes of data involved, AI needs vast amounts of processing power. The Economist reports that between January and March 2024, Alphabet, Amazon, and Microsoft collectively invested around $40 billion in data centers, specifically to deal with expanding AI workloads.
Most smaller and private data centers simply don’t have the power density for AI servers, so enterprises that are serious about AI innovation should be looking to hyperscalers. These are massive, highly-efficient, custom-built computing facilities that are designed for scalability and the high-speed processing of vast data volumes. Consisting of millions of servers, cooperating in a distributed architecture, hyperscalers have the processing power necessary to make the most of applications like cloud computing and AI.
2. Network and cloud connectivity
In addition to servers, power, and cooling space, which have traditionally been their focus, data centers are increasingly offering advanced interconnection services. To make AI work effectively, enterprises need data to be able to travel between networks and cloud environments as quickly as possible via short, secure pathways that ideally avoid the unpredictability of the public Internet.
Enterprises should opt for colocation data centers that house or provide direct access to neutral interconnection platforms such as Internet Exchanges and Cloud Exchanges. With this type of interconnectivity it’s possible, for example, to privately route the data that’s being used to train your AI algorithms between different cloud environments within a single data center campus.
3. Geographic separation and localization
Diversity, neutrality, and redundancy are vital to building the resilient interconnectivity that will support AI applications. This is particularly relevant when it comes to data centers. It’s easy to see how a natural disaster or geopolitical disruption in a particular part of the world could have an outsized impact on operations if an enterprise depends too heavily on a single data center. To make best use of AI, businesses need redundant access to cloud onramps from geographically separated data center locations.
Of course there are other benefits to localized data centers beyond resilience. Having data centers geographically close to where the AI application is deployed helps to reduce latency, which could be critical for use cases like remote patient monitoring in healthcare or autonomous manufacturing. In addition, localization means data can be kept within specified geographic boundaries to help comply with evolving data regulations.
Learn more secrets to AI success
A robust data center strategy is just one of the elements your business needs to make the most of AI in the cloud. Read our guide, The Secret to AI Success to find out what your business needs to support AI innovation and put those incredible productivity gains well within reach.